Flight Missions 2012/2024 to combat illicit cannabis cultivation in Albania. Cooperation agreement among:
General Command of the ‘Guardia di Finanza’, Benecon University Consortium, SCIP of the Italian Interior Ministry, Albanian Government and Police. Mapping and creation of a database of the Albanian territory through airborne surveys with hyperspectral and very high-resolution photographic sensors to contrast illicit cultivation of cannabis.
Benecon metodology
The hyperspectral remote sensing activities in Albanian territory carried out from 2012 to 2024 were focused on the discretization of illicit cannabis plantations, hidden due to environmental orography or inaccessibility of places. Specific and targeted hyperspectral aerial remote sensing campaigns with the ITRES CASI-1500 sensor (from 2012 to 2021) ITRES uCASI-1920 sensor (from 2021-2024), suitable for recording the electromagnetic ‘responses’ of the natural and built environment in the segments of the electromagnetic spectrum ranging from 365 to 1050 nanometers. The appropriately processed data return ‘deep’ images of the natural and built territory structured by multiple spectral layers, which, appropriately classified, return thematic maps regarding: land use, vegetative stress of plants and crops, geological anomalies, botanical pollution, artificial soil materials, water pollution, just to mention the main critical applications.
ANALYTICS DATA 2012-2023
The sources of documents can be found in public presentations at the annual conferences held in Albania, promoted by the Albanian Government, the Ministry of the Interior – Albanian Police, by the Italian Ministry of Interior – SCIP , by the General Command of the Guardia di Finanza.
Also, the copious publicity is found in the Albanian and Italian media.
2.500 Hours for the preparation of missions’ flight plans and post processing relating to the use of technologies, software and algorithms of the Benecon University Consortium.
14.400 Hours committed in Albania by Researchers of the Benecon University Consortium for the analysis, interpretation, management and representation of data.
11.000 Hours for the integration and management of Big-Data implemented and integrated annually at the end of each mission by the Researchers of the Benecon University Consortium, in the laboratory, for the verification of the territorial scenarios represented and for the hypothesis of predictive scenarios of artificial intelligence taken by Machine Learning algorithms and dedicated software useful for testing and legitimizing the activities carried out and for the preparation of new operational scenarios on national and international territories.
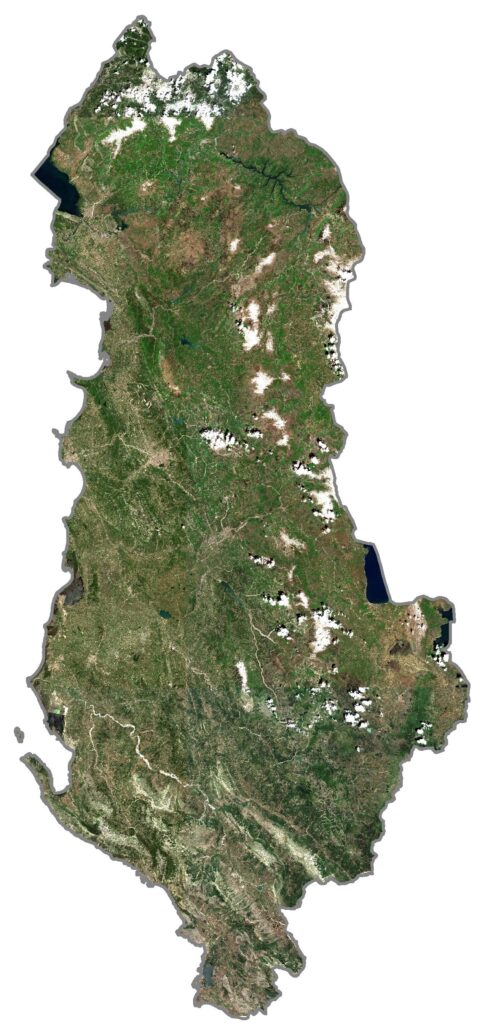
Image elaborated of Albania territory by Benecon University Consortium, source: Sentinel – 2 Satellite.
AUTOMATIC IDENTIFICATION OF CANNABIS PLANTATIONS BY MACHINE LEARNING AND DEVELOPMENT OF FORECAST SCENARIOS TO SUPPORT DECISION-MAKING PROCESSES IN THE ACTIVITY OF CONTRASTING CANNABIS CULTIVATION
The machine learning algorithms are organized in a taxonomy based on the desired result of the algorithm; then a function is generated that maps the assigned inputs to the desired outputs.The generation of large volumes of data (Big Data) has made machine learning techniques highly sophisticated. This required the use of different algorithms for machine learning both of the supervised and unsupervised territory. Supervised learning is necessary for Big-Data classification and management activities because the aim is to make the computer learns a classification system that we have previously developed by labeling the inputs with some labels (identified hot spots). A standard formulation of the supervised learning task is then represented in a classification scenario: the algorithm must learn how to approximate the behavior of a function that maps a vector into one of two different classes (cannabis / no-cannabis), examining different spectral traces. Inductive machine learning is based on a learning process based on a set of rules drawn from exemplary protocols (examples present in a training set) or, more generally, on the creation of a classifier that represents the starting point for new instances.
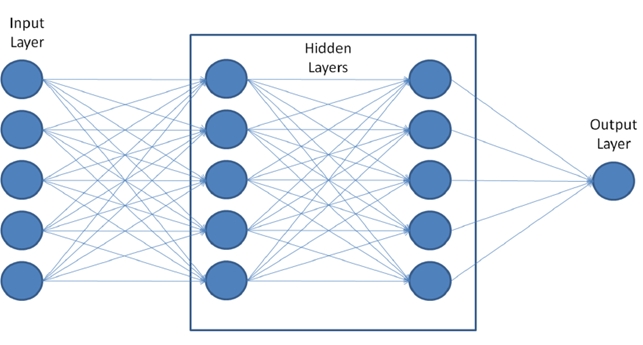
Architecture of an artificial neural network with two hidden layers (cannabis / no-cannabis).
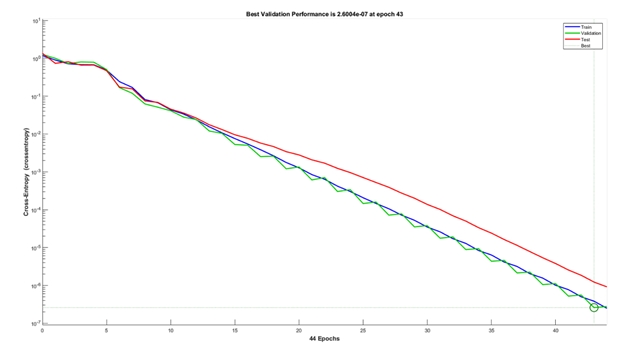
Error trend during the training process.
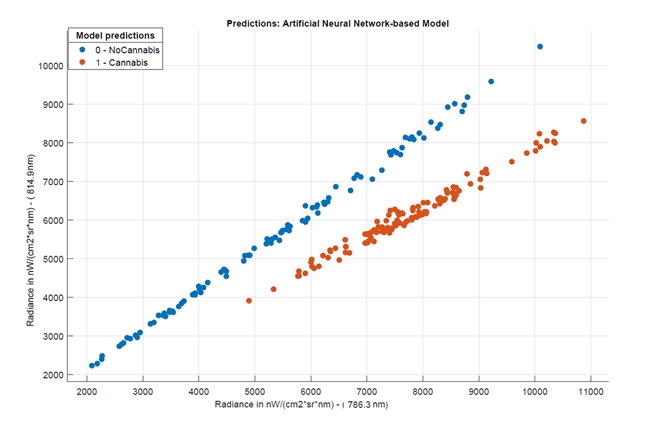
Distribution of the classifications in the two-dimensional plane identified by the two spectral components that turned out to be priority in the classification of the model (786.3 nm, and 814.9 nm) used.